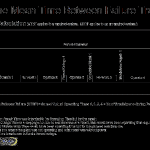
An MTBF calculation is often done to generate an indicator of plant and equipment reliability. An MTBF value is the average time between failures. There are serious dangers with the use of MTBF that need to be addressed when you do an MTBF calculation.
Take a look at the diagram below representing a period in the life of an imaginary production line. What is the MTBF formula to use for the period of interest to represent the production line’s reliability over that time?
If MTBF is the ‘mean time between failure’ (MTBF applies to repairable systems; MTTF, Mean Time To Failure, applies to unrepairable systems) the MTBF formula would need to have time units in the top line and a count of failures on the bottom line.
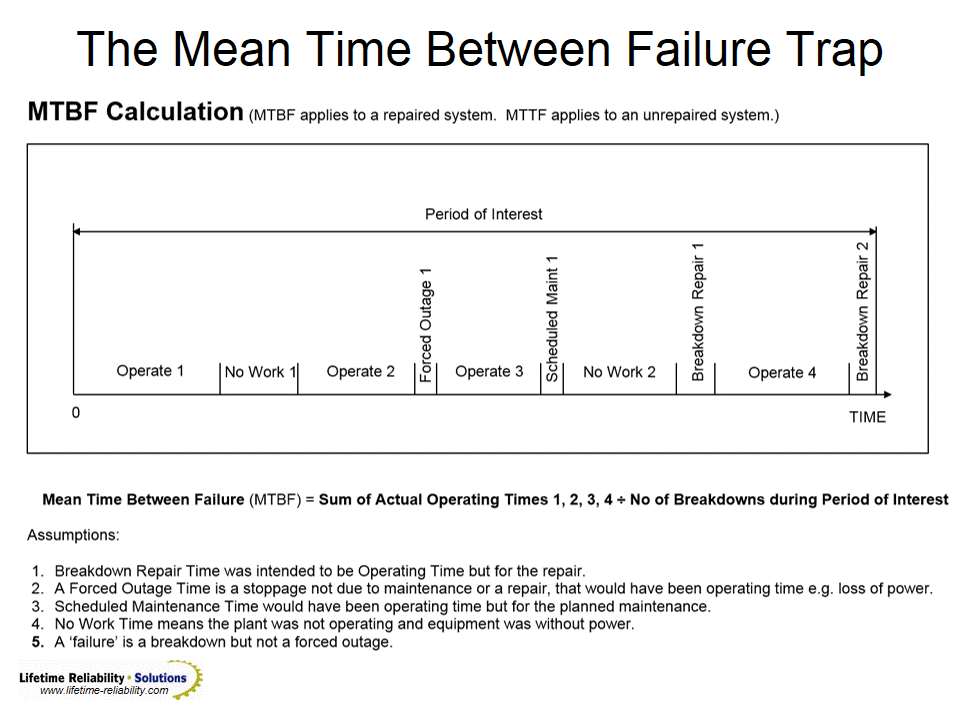
In the diagram you will see the MTBF formula that I finally settled on: Mean Time Between Failure (MTBF) = Sum of Actual Operating Times 1, 2, 3, 4 divided No of Breakdowns during Period of Interest.
But the MTBF value you get from that MTBF calculation changes depending on the choices you make.
To arrive at a MTBF equation there are assumptions and options to consider. Like, what event is, and is not, a ‘failure’? What power-on time do you consider to be equipment operating ‘time’? When do you start and end the period of interest for which you are doing the MTBF calculation?
Definition of Failure
To measure MTBF you need to count the failures. But some failures are out of your control and you cannot influence them, like lightning strikes that fry equipment electronics, or floods that cause short circuits, or if your utility provider turns off the power or water supply. Do you include Acts-of-God into your MTBF calculation?
In reliability engineering a ‘failure’ is considered to be any unwanted or disappointing performance of the item/system being investigated. That definition leaves ‘failure’ wide-open to interpretation.
Is a ‘failure’ only ever a breakdown? Is a ‘failure’ anytime the production line stops no matter the cause? Is a power black-out caused by the utility provider a ‘failure’ you should count? Is an operator error that stops production but does no other harm a ‘failure’? Should you include all types of failures in your MTBF calculation—that will give you a short MTBF value? Or do you remove certain categories of stoppages when using a MTBF formula—that will give you a longer MTBF value? But which categories do you and don’t you count?
If in the imaginary production plant timeline modelled above you included ‘Forced Outage 1’ along with the two breakdowns in the MTBF calculation you would get a MTBF one-third lower. That is a substantial impact on the MTBF value.
To make sense of a MTBF calculation you need to know what ‘failures’ are included and which ‘failures’ are not. And you also need to understand why those choices were made.
Definition of Operating Time
When is an item of plant or equipment operating?
Equipment parts are degraded by the applied stresses put on their atomic structure. The greater the stress suffered, the greater the resulting impact on the item’s operating life. When a vehicle is stopped at red traffic lights the engine is running under the least working load. The gearbox and the rest of the drive train are not in use. When parts are under no stress their atomic structure suffers no harm. When equipment working assemblies are at least stress their parts last longer. For the MTBF calculation of the vehicle do you include its idle times, or just the times it carries sufficiently high working load that causes stress in the parts?
Would you consider the ‘equipment operating time’ for the MTBF calculation as any time it was turned on, or only when it was suffering under working loads? If in the MTBF formula you included all operating time from when the vehicle started, and not only when the parts were under working stress, your MTBF value would be higher. But that Mean Time Between Failure value would not be representative of those vehicles that are continually working and hardly ever idling.
You cannot use MTBF as an indicator to compare the same equipment model, assembly number or part number if they are suffering under different working situations.
To make sense of a MTBF calculation you need to know the specific situation and operating scenarios being measured.
Selecting the Time Period
Because you count ‘failure’ events during a period of time in your MTBF calculation the period of interest selected affects the resulting MTBF value.
In the above timeline the period used in the MTBF reliability analysis is through to the end of the second breakdown. If I had chosen to make the time period through to the end of ‘Operate 4’ the second breakdown would not be counted in the MTBF calculation and I would double the MTBF value. By altering the date to exclude one failure event I doubled MTBF—see what magic you can do with MTBF.
Notice how the two equipment breakdowns are well to the right hand side end of the timeline. Even though the first breakdown happened long into the period of interest, the MTBF for the period does not recognise the dates of those failures. The MTBF was outstanding performance up until the first breakdown, then it dropped, and it dropped again with the second breakdown. A MTBF calculation presumes ‘failures’ are distribute evenly across the period, even though that is not the real historic truth. A MTBF value is hardly ever honest about what actually happened.
To make sense of a MTBF calculation you need to know the time period selected. You also need to know why that duration was used and not some other period.
Selecting the Equipment to Monitor
One more issue to consider with regards MTBF is whether you measure a whole process or measure individual equipment within a process. A complete process suffers MTBF loss every time one of its critical items ‘fail’. If you have a problem piece of plant that brings down the MTBF performance of the whole process, the ‘bad actor’ needs to be flagged as the performance destroying cause.
The companies who take the whole line/process into MTBF calculations often struggle to get a high MTBF due to ‘bad actors’ failing within the system being monitored. Those companies also need to measure individual equipment MTBF to identify the problem plant so its failure causes can be addressed and the ‘bad actor’ made more reliable.
How to Protect Yourself from the MTBF Calculation Trap
MTBF calculations are a statistical trap easily fallen into. A MTBF value can be a total fabrication. Some Managers remove or add all kinds of MTBF parameters to make their department look good (like not counting ‘failures’, changing period lengths, and the like). But that is a falsehood. I once came across a company that did not count stoppages less than 8 hours duration in their MTBF calculation. They weren’t ‘breakdowns’ but they were forced outages over which they had full control. What a joke I thought. What an absolute rubbish way to run a business. You can never improve a company if people tell lies about its performance and hide the truth of where the troubles lay.
You need to get agreement across the company as to what can be called a ‘failure’, what can be called ‘operating time’ and what are the end points of the time period being analysed before you can use MTBF values as a believable Production Reliability KPI (Key Performance Indicator).
Maybe it is more sensible to have MTBF by categories, e.g. 1) mean time between machinery/equipment breakdowns caused by internal events, 2) mean time between operator induced stoppages, 3) mean time between external caused outages that you cannot control, like power or water loss, and so on.
Your second best protection against misinterpreting and misunderstanding MTBF is to have honest, rigid rules covering the choices and options that arise when doing a MTBF calculation.
The very best protection is to also get the timeline of the period being analysed showing all the events (and their explanations) that happened, and then ask a lot of questions about the assumptions and decisions that were made, and not made, to arrive at those MTBF values.
All the best to you,
Mike Sondalini
Managing Director
Lifetime Reliability Solutions HQ
Great work going into deep details of how MTBF is best measured, and explaining the false statistics we have seen applied before. At least you did not put down the concept of using MTBF as a reliability indicator; It is not perfect but it is the best available reliability measurement criterion regarding Machinery or Instrumentation, Electronics, and Electrical devices.
Hi Abdulrahman, please consider the reliability metric very suitable. A probability of failure over a specific duration with associated function and environment. We can estimate or calculate the reliability metric using a wide range of parametric and non-parametric methods.
I agree with Mike in this article – all too often the MTxx values provided by vendors or even shared within an organization are less then useful even if calculated correctly and consistently. We are not interested in an average value that inherently makes major assumptions related to a constant hazard rate for our equipment. MTxx values rarely, very very rarely reflect the actual pattern of failures.
We should use the best available data, that may take a little more work and better understanding compared to using MTxx value, yet provides a means to make better decisions.
cheers,
Fred