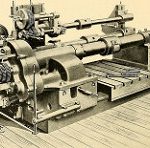
What About Weibull? Can I Use it Instead of MTBF?
This was a follow-up question in a recent discussion with Alaa concerning using a metric other than MTBF.
The term ‘Weibull’ in some ways has become a synonym for reliability. Weibull analysis = life data (or reliability) analysis. The Weibull distribution has the capability to describe a changing failure rate, which is lacking when using just MTBF. Yet, is it suitable to use ‘Weibull’ as a metric?
What To Use Instead of MTBF
Use reliability, which is the probability of successful operation over a defined duration. This typically includes a defined environment as well.
It is the definition of reliability, as we use it in reliability engineering.
Instead of saying we want a 50,000-hour MTBF for the new system, we should say we want 98% to survive 2 years of use without failure.
Be specific and include as many couplets of probability and duration as are necessary and useful for your situation. For example, you may want to add that 99.5% survive the first month of use and 95% survive 5 years of use.
Weibull is a Distribution, One of Many
Weibull, Lognormal, normal, exponential, and many others are names of statistical distributions. They are formulas that describe the pattern formed by time-to-failure data (repair times, and many other groups or types of data).
Instead of Weibull Analysis, you could easily say we’re going to conduct a Normal analysis. In reliability work, I often first explore a set of life data by fitting a Weibull distribution to the data and plotting the probability density function (PDF) and cumulative density function (CDF). It’s a first look, not the end of the analysis.
Each distribution has four functions that are useful for reliability engineering work:
- Reliability function
- Cumulative density function
- Probability density function
- Hazard function
Since I tend to like being positive about a product, I often use the reliability function (calculated at specific points in time, t) instead of the CDF which is the probability of failure over time, t.
The reliability function is a function of time, so I suggest always including probability and duration when specifying or reporting reliability values.
Weibull is a Distribution, Not a Metric
Like other distributions, the Weibull distribution is a curve or equation. It is not a metric on its own.
Define the time intervals of interest, run out the calculations (I recommend using the reliability function for the appropriately fitted distribution), and then you will have a metric.
Goals are not metrics but should be something we can measure and help us make better decisions. For example, setting reliability goals for one month, the warranty period, and over the expected use life.
Then, use vendor or testing data and/or field data to estimate the distribution of the life data. Then again, for specific time intervals of interest, calculate reliability. Now, you can compare your data to your goals and make informed decisions.
Just doing ‘Weibull’ is not a metric.
In many circumstances, it is clear that when someone says they are going to do a Weibull Analysis, it is really a life data or reliability analysis not limited to fitting a Weibull distribution—at least, I hope so. The result of the analysis may be an estimate of reliability over a time period of interest.
How do you use the term ‘Weibull’, or how have you heard it misused? Add your thoughts or observations in the comments below.
Hi Fred,
Nice post on Weibull.
But it does not end here, choosing a distribution is only the first part (there are tools to help you with that)
But we have to take in account other factors like:
– do we have enough samples to be statistical relevant?
– use median rank regression, MLE or something else (when talking Weibull)
And than again the metric issue…
Can you show us in an example how you would do it?
Using a Weibull metric.
I can show improvement in my design by Weibull, but how to use these metric between different kind of designs, or can’t we?
The next hurdle will be to convince the suppliers to provide Weibull based data (or better: life data)
Thanks for the comment Tim – and you are right there is more to this ‘weibull’ analysis approach and more to metric. Sounds like you may have an article or two to write. cheers, Fred
I have seen many engineers using Weibull to determine if a set of failure data represents infant mortality, random failure with constant failure rate or wear out. The problem is that they get the data from a complex equipment without taking care about failure modes. I mean, they do not separate the data into different failure modes. Is it a correct approach? All of them useed Reliasoft Weibull Analysis software.
Thanks for the comment William – I agree that just fitting and plotting without considering the data source and nature is a problem. cheers, Fred
Accounting for several failure modes can be done but it requires some mathematical care and a lot of good data, both of which are hard to come by.
😉
By the way: if you do Weibull analysis of a large system, the result will tend to an exponential because you are averaging over many failure modes with various time scales for failure.
http://www.bqr.com/bqr-releases-field-data-analysis-tool-for-actionable-information-on-spare-parts-and-maintenance/
Hi Amir, careful with the notion that you can assume exponential with a large system. If there is one or two dominating failure mechanisms then the averaging doesn’t apply. If all the wear out mechanisms are happening at the same time, like my first car, then the averaging doesn’t apply. If the system is new and poorly maintained then the averaging doesn’t apply.
Always, always check the assumptions concerning the use of exponential which also takes mathematical skill and enough data.
Cheers,
Fred
Hi Fred,
I agree, you always have to be careful and aware of assumptions.
My claim is not that you can blindly approximate distributions as exponential.
I was merely referring to Palm–Khintchine theorem.
I had some cases where indeed Weibull analysis of a large system gave a shape factor close to 1 whereas for the sub-components the shape factor was quite different.
Cheers,
A
Hi Amir,
The theorem is great in theory, yet we can only impact smaller systems or elements when working to improve reliability. Thus the theorem tends to provide more confusion, false analysis, and poor assumptions than helps anyone do anything useful.
It’s still not ok to assume exponential, even in a large complex system, as it is less then helpful.
At least that his the way I see it.
Cheers,
Fred
Hi Fred,
Very nice article. yea, I agree with Tim about the scarcity of data to arrive such a specific survival rate within a specific period.
thank you,
One of the perils of being a statistician we always want and need more data.
It’s not the same.
MTBF is applicable for repairable system; a Weibull analysis is for non-repairable item.
Adrien,
MTBF is for repairable systems, considering that the failure pattern follows exponential distribution (constant failure rate), which hardly ever happens.
Right, but what happen if the failure rate (hazard rate indeed) is not constant?
For example have a look at the Crow-AMSAA model, where the instantaneous MTBF is function of time : MTBF(t) = 1/ ( a.b.t^(b-1) )
If the failure rate is not constant, MTBF has no meaning. By using Weibull we can determine if that set of data represents infant mortality, constant failure rate or wear out. Then you apply the correct proactive maintenance strategy. But, to be effective the data must refer to a particular failure mode.
Hi,
I use a weibull esimation for my data, and I want to get the “MTBF” or something similar from the Weibull is it possible ?
take a look of my question in stackoverflow, thanks
https://stackoverflow.com/questions/53585547/weibull-theory-with-r-mtbf-calculation-for-censors-data
Hi Mostafa,
Not sure why you or anyone would want to calculate the mean of a Weibull distribution, yet you can. See http://nomtbf.com/2015/05/determine-mtbf-given-weibull-distribution/
Cheers,
Fred
One of the reasons companies use MTBF is because their suppliers talk the same language. Many suppliers would come back and say “sorry we dont know what you are talking about” if they were asked to provide reliability 99% in x hours. Majority of the suppliers I interacted with know only MTBF/failure rate.
What was your experience on this?
Hi Ayaz,
As you know, MTBF and related measures are a very poor reliability metric for many reasons. Instead of settling for vendors that only wish to provide MTBF and nothing else, my first thought is to get a new vendor that cares about the reliability performance of their product. In reality, this is not always an option, so I strongly suggest engaging your vendor in a better discussion about their product’s expected reliability and performance in your system.
The idea is to share with the vendor how you are using their product (environmental conditions and use rate, etc.) Then ask them how they expect their product to fail in such conditions. Get specific failure mechanisms. They ask how they know that information, ask for supporting data or information. From there you certainly can have a meaningful discussion about when to expect the first 1% of units to fail, etc.
I have found the sales folks tend to believe their product will never fail in anyone’s system – basically, find someone in the organization that understands the product, technology, and limitations.
I did a webinar on this topic – https://fred-schenkelberg-project.prev01.rmkr.net/accendo-courses/accendo-reliability-webinar-series/lessons/suppliers/topic/asking-a-vendor-for-reliability-data/
cheers,
Fred