
When Weibull analysis is applied to complex, repairable systems – like mining equipment – care must be taken to ensure the analysis is applied to failure data exhibiting a common failure mode on the component-level, not the system-level.
This requires the reliability engineer to review the work order data at times and, ideally, the failed components themselves. Otherwise, the “Garbage In, Garbage Out” principle applies.
It is relatively well known in the reliability engineering community, that multiple failure modes in a simple Weibull analysis will give S-curves, dog-legs and in general a very poor fit to the failure data. This is because different failure modes will each have their own unique failure characteristics and as such their own Beta values and characteristic life (Eta). (this article does not cover multi-modal Weibull analysis where the failure modes are dependent).
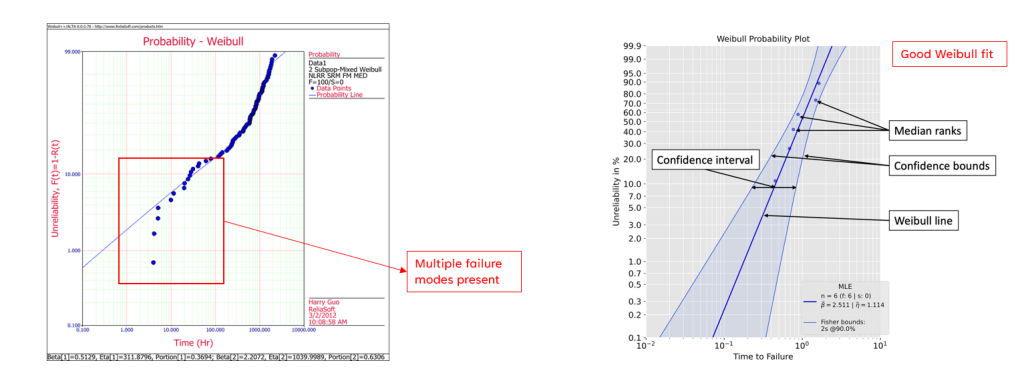
Experience will tell us to trust the wellness of fit from the Weibull plot, and take caution when the fit is poor, even when you think your data is reliable.
This brings me to an example I experienced during some consulting work a while back…
A Reliability Engineer at one of my client sites contacted me about the strange results he was getting for his Pump replacement time- 2100 Hours! Normally for this particular pump we would expect 12K hours.
The Beta value from his Weibull analysis was of a value slightly higher than 1, indicating slight wear-out characteristics, but this final replacement interval calculation gave a quite unreasonable value. I dug into his model, and it turns out his calculations were correct, however the method of classifying the data was flawed.
The life data collected from SAP was simply the previous replacement hours/ pumps still running in suspension using the part counter reading, which normally is correct, however it turns out that the field maintenance workers would simply replace the full pump with a new one when it broke down, with various failure modes noted in the Work Order itself. These failure modes ranged from bearing failure, case wear-out to impeller damage due to cavitation. These failure modes were not separated into individual Weibull Analyses, and a multi-modal model couldn’t be applied as the failure modes were independent.
Mixing of failure modes of varying shape parameters in a single Weibull analysis will often return a shape parameter around 1, leading to the assumption of a random failure distribution. This phenomenon is known as “Drenick’s Law” (F. Drenick, R. (1960): The Failure Law of Complex Equipment; Journal of The Society for Industrial and Applied Mathematics.).
Although in this scenario, it was quite obvious that the hours were wrong and we were able to fix the issue, there are many component strategies out there in which the hours-based strategy doesn’t raise any eyebrows, yet has the same flawed basis as this case, leading to unnecessary costs down the track.
Leave a Reply