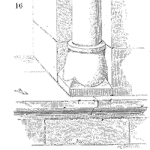
Modeling Complex Systems and Their Variability
Monte Carlo relies on data that describes the variation of elements within the system. It also connects the elements such that they result is an estimate of performance.
For reliability modeling, this is easiest to imagine for a series system.
For a system with two elements in series, a very simple reliability block diagram multiples the expected reliability for each block to determine the system reliability value. Yet, it is possible to have both elements at the low end of the range of possible reliability values, or the high end or a mix.
That is the value of the Monte Carlo approach.
For a simple series model with two elements, and if we have the Weibull function describing the reliability as a function of time for both elements, plus the range of uncertainty for the Weibull model.
So, for example, at one year the reliability of the first element may range from 90% to 95% with a mean of 93%. Then we can randomly select a reliability value for each element from their respective distributions and then multiply to find the system reliability value.
Of course, the mean values provide an estimate of the estimate mean system value.
If that is all you need then you do not need the Monte Carlo approach.
If you want to estimate the range of system values that the system is likely to produce, or the distribution of possible system reliability values, then you can use Monte Carlo.
When Use Monte Carlo for Modeling?
For two elements in a system it is possible to determine the system reliability mathematically by combining the two distributions, yet for a complex system with many variables impacting the system reliability of any one system, then a Monte Carlo approach may be an efficient solution.
A clear case when Monte Carlo works well is when there is a combination of environmental, user or usage, and component variables that impact the system performance. For example, if a portion of your products are in a dry cold environment they would see a very different rate of corrosion related failures than those in hot moist environments.
Even if you only have rough percentages of units in different environments, that allows the model to improve its ability to estimate the overall fleet system reliability expectations.
Why use Monte Carlo?
You need accurate system reliability information to make decisions.
The Monte Carlo approach takes more data and a bit of work to create, yet can provide the necessary accuracy when estimate system performance.
An added benefit of the approach is the need to gather and understand the variability that affects the system reliability performance. The initial models may have only crude estimates of the necessary variables, like using just hot and cold for temperature. Yet, it highlights the need to understand the temperature variability and it’s relation to system reliability though it’s impact of failure mechanisms.
Thus, the team gathers two kinds of information: Environmental temperature data that describes the distribution pattern of temperature in different regions, plus the failure mechanism models that relate time to failure given temperature. Both help to improve the overall system model.
Another benefit is the ability for the team to conduct ‘what if’ analysis at a finer granularity. Like a sensitivity analysis, the model can help the team understand which set of variables and sources of variation causes that greatest impact on system reliability. This is handy when working to achieve a consistent system reliability performance.
Give it a look and start building even a simple model. How do you use Monte Carlo and has it made a difference for your team?
For an example of Monte Carlo in use for tolerance analysis, see the article, Reliability and Monte Carlo Determined Tolerances
Can you show a practical example of this? Say an Excel spreadsheet to download and understand an implementation.
Sure, check out the webinar recording at https://fred-schenkelberg-project.prev01.rmkr.net/accendo-courses/accendo-reliability-webinar-series/lessons/data-analysis/topic/using-monte-carlo-simulation/
Where Chris builds a MC model and provides the spreadsheet as a download.
cheers,
Fred