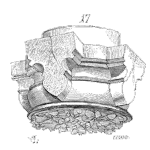
A parametric Life Analysis involves “forcing” or “imposing” a distribution’s parameters on a data set in order to obtain the “best fit”. However, it can lead to errors in results. The non-parametric estimation suggests that there are other approaches though not necessarily the easiest or “most elegant” ones. In the field of reliability engineering, we tend to like something so much that we use them in every “sauce”. A classic example is the Weibull Distribution. It has become so popular that Life Analysis is also known as a “Weibull Analysis”. As a reminder, the Weibull distribution is only one parametric distribution amongst a myriad of others, invented by Walodi Weibull in 1937. Dr Bob Abernathy’s New Weibull Handbook1 quotes: “the Weibull distribution provides reasonably accurate failure and failure forecasts……”. Thus, parametric distributions are good enough but not perfect to make a decision.
As a student of Chemical Engineering, professors always warned us of the following. Any measuring device used to measure the parameters of a microscopic entity should not interfere with the latter’s state. In other words, will your testing devices end up altering your results? In essence, the method we are using to estimate life performance should not lead us to incorrect results. By imposing a distribution on the data set, we run the risk of getting the wrong results.
Larry George in an Accendo article summarizes this concept in a different yet very pertinent way. “Data is inherently non-parametric”. “Reality is more interesting, but quantifying reality takes work…” leading us to default to the “mathematical convenience” using parametric estimations.
The “Elegance” of Parametric Distributions
Parametric distributions provide us with a large amount of information and this is why we tend to use them. For example, the two parameter Weibull distribution can provide us with the following:
- A Bath-tub curve, rich in information on the life cycle of the asset studied,
- The shape parameter (beta) provides information on life evolution in the form of failure rates,
- The scale parameter (eta) provides a quick indication of the probability of failure (63.2%) at this value. Not far from the median (50%) value so we can roughly gauge the remaining life of the asset,
- The Cumulative Density Function can easily be transformed into a straight line through a logarithmic transformation. Engineers happen to like straight lines,
- The Weibull distribution parameters can be calculated manually, on paper, using logarithmic scales,
- And it goes on….
As a result, parametric distributions provide a lot more information about an asset studied. They can help with a variety of decisions. Hence their popularity.
Worked example of parametric and non-parametric estimation
The following example is based on 34 gearbox systems. 10 failures occur during the recorded interval. Gearboxes are repairable systems. The General Renewal Process (GRP) is applied. Graph 1 below provides a comparison of the two cumulative failure estimations over time.
One can clearly see that the parametric estimation has a more streamlined curve fit. Additionally, the Weibull distribution and derived parameters (shape and scale) provided indicate information such as:
- A shape parameter (beta) lower than one suggesting that failures recorded have infant mortality characteristics
- An estimated characteristic life for a gearbox of 741,694 hours (or 85 years!). This is a lot of years! One has to be careful with this interpretation as those gearboxes have been tested for a maximum of 3 years. Longer periods of testing are required to refine this calculation. This is where the “engineering oversight” comes versus just calculating numbers.
- The above two points indicate that the gearboxes tend to fail early in their life cycle. This suggests deficiencies in the manufacturing process or installation.
- The Restoration Factor (RF) is also available and equal to zero suggesting that any repair restores the gearbox system to as “good as old”. In other words, repairs to a failed gearbox are not very effective.
Conversely, the non-parametric estimation is not that informative. The only practical information that we obtain is the estimated number of failures over time.
A further comparison between the two methods of estimation is provided below. Table 2 provides the comparative cumulative number of failures at time 8,250 hours (344 days) post install.
The non-parametric estimation is more conservative predicting more failures during a specific time interval after install. Its coefficient of variation value is lower indicating more confidence in the model estimation.
In summary, even though the parametric model provides us with more information than the non-parametric one, it is important to note that the latter is closer to reality. The analyst should take note of the difference. Unless one is designing expensive and highly sensitive equipment like satellites, it is acceptable to use parametric estimations.
- Abernathy, Robert B., “The New Weibull Handbook – 5th Edition, Section 1.5, pp 1-3, 2004
The data came from https://fred-schenkelberg-project.prev01.rmkr.net/graphical-analysis-of-repair-data/#more-182 Wayne Nelson’s article. Thanks André-Michel for sharing the data with me. The failures seemed premature infant mortality, and Weibull fit nonparametric reliability pretty well. However, the nonparametric estimator and its Weibull fit quantify infant mortality but do not take advantage of all the survivors’ lifetimes.
Thanks Larry for collaborating on this one. Always a pleasure to work and learn with you. I did find the Article you quote in the New Weibull handbook – Appendix M3 specifically. There seems to be some similarities between the one I quoted from the Reliasoft software. Reliasoft quotes time to failure whilst Nelson uses miles to failure. I reproduced the example in the Supersmith software which I have. I had never used the “Nelson Calculator” before in Supersmith.