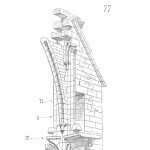
A one-sample t-test is a statistical test to determine if the sample mean differs significantly from a known or hypothesized population mean (expected value). It is commonly used when we have a single sample and want to compare its average to an expected value.
Steps of One-Sample T-Test
1. State Hypothesis
- Null ($-H_{0}-$): No difference between sample mean and population mean ($-H_{0}: \mu=\mu_{0}-$)
- Alternative ($-H_{a}-$): Difference between the sample mean and population mean ($-H_{0}: \mu\neq\mu_{0}-$) for a two-tailed test)
2. Sample Mean and Standard Deviation Calculation
Sample Mean ($-\bar{x}-$) represents the average value of the sample, and the sample standard deviation ($-s-$) measures variability/spread within the data.
3. Compute t-Statistic:
t-statistic measures how far the sample mean is from the population mean, scaled by standard error. The formula is:
$$ t=\frac{\bar{x}-\mu}{s/{\sqrt{n}}} $$Where: $-\bar{x}-$ = sample mean
$-\mu-$ = population mean
$-s-$ = sample standard deviation
$-n-$ = sample size
4. Degrees of Freedom
- Degrees of freedom ($-df-$) are calculated as $-n-1-$. Using a t-distribution table or software, we can find critical t-value for the chosen significance level (default = 0.05)
5. Insights and Conclusions:
- If calculated t-value exceeds critical value (p value < 0.05), null hypothesis is rejected, indicating statistical significance.
Reliability Examples using One-Sample T-test
In reliability analysis, a one-sample t-test can be used to evaluate whether the average lifetime or failure rate of a component or product meets specific target per design specification or industry standards.
- Mean Time Between Failures (MTBF): A one-sample t-test helps to determine if observed MTBF is significantly different than manufacturer specifications helping team to assess whether components meet reliability expectations.
- Failure Rate: By performing one-sample t-test to compare measured average failure rate of a component from reliability test results versus expected failure rate, companies can verify if sample performance aligns or diverges from the expected rate, which is critical for meeting quality, reliability and safety standards.
Source: JMP, Machine Learning Plus
Leave a Reply