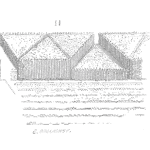
The McNemar test is a nonparametric statistical test to compare dichotomous (unique) results of paired data.
If you are comparing survey results (favorable/unfavorable) for a group of potential customers given two ad campaigns, or evaluating the performance of two vendors in a set of prototype units, or determining if a maintenance procedure is effective for a set of equipment, this test permits the detection of changes.
The McNemar test is similar to the χ2 test. The McNemar only works with a two by two table, where the χ2 test works with larger tables. The χ2 test is checking for independence, while the McNemar test is looking for consistency in results.
Let’s examine an example where a group of people are surveyed about a prototype design, before and after a presentation.
The Question
We want to know if the presentation is effective in changing people’s mind about their opinion concerning a new product concept.
Some people in the group will be favorable before and after the presentation, and some will have an unfavorable opinion before and after the presentation. Some will change their opinion, randomly, and some will or could be influenced by the presentation. We want to know if the number of people that changed their stated opinion is more than expected by random chance alone.
The Assumptions
#1 The categorical dependent variable has two categories and are dichotomous (distinct and unique), and there is one categorical independent variable with two related groups (before/after, pretest/posttest, matched pairs, or a treatment group and control group study. The pairs are mutually independent.
#2 The results (favorable/unfavorable, yes/no, etc) must be mutually exclusive, meaning the in this case someone cannot be both favorable and unfavorable at the same time.
#3 The sample of people, in this case, or of subjects in general, is a random sample from the population of interest.
The Test Setup
The first step is to arrange the data into a 2×2 contingency table.
Let’s say we have 145 people that we survey about a product concept and 117 are favorable and 21 are unfavorable. We then present additional information and details to the group and conduct the survey again. After the presentation, there are 100 people favorable and 45 unfavorable. Was this chance or an indication of a real shift in opinion?
Favorable After Presentation | ||||
Yes | No | totals | ||
Favorable Before Presentation |
Yes | 79 (a) | 38 (b) | 117 |
No | 21 (c) | 7 (d) | 28 | |
totals | 100 | 45 | 145 |
The null hypothesis is the number of people changing their opinion from favorable to unfavorable equals the number of those switching from unfavorable to favorable.
The alternative hypothesis is the number switching opinions is not the same in the two directions.
The Test Statistic
The McNemar test uses the two discordant cells (the two cells that have a change, in this case from favorable to unfavorable and vice versa.) The cells marked (b) and (c) are the two cells we are interested in evaluating.
The McNemar test formula for the test statistic is:
$$ \displaystyle\large {{\chi }^{2}}=\frac{{{\left( b-c \right)}^{2}}}{b+c}$$
Adding the numbers we have
$$ \displaystyle\large {{\chi }^{2}}=\frac{{{\left( 38-21 \right)}^{2}}}{38+21}=\frac{441}{59}=7.47$$
McNemar uses the χ2 distribution to evaluate the magnitude of the differences in the discordant cells.
The Critical Value
The McNemar test has one degree of freedom and we are interested in detecting a shift with 95% confidence. C = 0.95 thus 1-C = α = 0.05, the level of significance. Thus the critical value is
$$ \displaystyle\large \chi _{1-\alpha ,1}^{2}=3.841$$
The Conclusion
The test statistic is larger than the critical value indicating the change in opinions is more than would be expected by random chance. The p-value, for those that like such values, is 0.0372, which is less than 0.05, again suggesting the difference of opinion from before to after the presentation is significant.
Just another tool for your stats toolbox. If you have used this test, add a comment on the circumstance and why you selected the McNemar test.
Leave a Reply