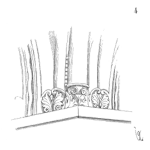
Definition of repairable versus non-repairable assets
A common error when performing a life analysis for an asset is to confuse repairable and non-repairable assets. The mathematical determination of the life characteristics for each model is different, so throwing a simple “Weibull analysis” at them might lead to the wrong results but also the loss of valuable information.
A repairable system is expected to fail multiple times over its life. It will be repaired and restored to as close as possible to its original functional state. However, it is very rare that it will be restored to an “as good as new” state because other parts of the system which have not failed yet are in a state of deterioration and will fail sooner or later. Subsequently, failures on this system will occur more and more frequently over time. A good example of a repairable system is an automobile. Changing a failed component such as the gearbox will get the car going again, but the rest of the original components such as the engine or drivetrain will retain the same amount of wear, making the whole automobile “as good as it was just before it failed.” Moreover, the shiny new gearbox might not last as long as it would in a new car; it will be subject to induced deterioration caused by the aging system around it and therefore fail sooner than expected. Therefore, when we talk about repairable systems, any repair conducted on the system brings it to an “as good as old” or a “minimal repair” state.
On the flip side, a non-repairable asset is only used once; it is typically a consumable such as a light bulb, a bearing, or a gasket. Replacing the light bulb in its original location makes the lighting system, in essence, “as good as new,” and the new light bulb is expected to last as long as the previous one.
Hence, mathematically speaking, repairable and non-repairable assets have to be treated differently when a study of their life cycle is conducted. If we treated the above-mentioned automobile as a non-repairable system, it would be expected to last forever as each repair would bring the whole automobile as good as new. As we all know, cars age over time due to collective wear. The same would apply to a pump or a valve.
Using the “restoration” or “renewal” factor
In order to model the failure process of repairable systems, the Non-Homogeneous Poisson Process (NHPP) is widely used. A key assumption of the NHPP model, in the reliability engineering context, is that upon a failure, the system is repaired to the condition it was in right before the failure. It also accounts for the reduction in failure intervals as shown in the diagram above, in other words, failures are expected to happen more frequently over time. One of the interesting benefits of using the NHPP process is an indicator known as the “restoration” or “renewal” factor, a number between 0 and 100%. This gives the operator an indication of how much of a “restoration” the repair has brought to the entire system. For example, if the restoration factor is 90%, this would mean that 90% of the initial life of the system was restored. It can also provide the maintenance department doing the repairs an idea of how “effective” their maintenance performance is. If it is below 100%, it could be to no fault of theirs but rather due to the system’s own characteristic life behavior.
The restoration factor is a key input in Reliability, Availability, and Maintainability models, which typically contain a large number of repairable systems such as pumps, valves, or motors. This number helps correctly evaluate the overall maintenance strategy of the operation in question (i.e., the “M” in RAM) and its precise influence on the output. Another benefit of the restoration factor is to evaluate the effectiveness of an equipment overhaul. There is a common myth in the industry that an overhaul brings the equipment to an “as good as new” state. Calculating this factor can confirm or disprove this assumption.
Good! I call that hysterecal reliability analysis when TBFs deteriorate (hysteresis). Want to estimate distribution of successive TBFs’ reliability functions from ships (installed base cohorts by age) and returns (complaints, repairs, failures, replacements, spares sales, etc.) counts, without life data? The biostatisticians love “proportional hazards” models, and the proportionality function quantifies the hysteresis. I used generalized renewal process model to make nonparametric reliability estimates for TTF1 and TBFs for automotive aftermarket parts where TTF1 is different from subsequent TBFs. Worked pretty well, especially for older cars. I’m looking for ships and returns counts or grouped failure counts to try out method for proportional hazard model.