
One of the common questions teams have when they first explore using Predictive Maintenance is “Is the data good enough to perform the analysis?” Answer to that question is nuanced with the reliability objective and the quality of the data available.
Before diving deep into the answer, a handy high-level view of the type of Predictive Maintenance analyses possible from available data is given in the table:
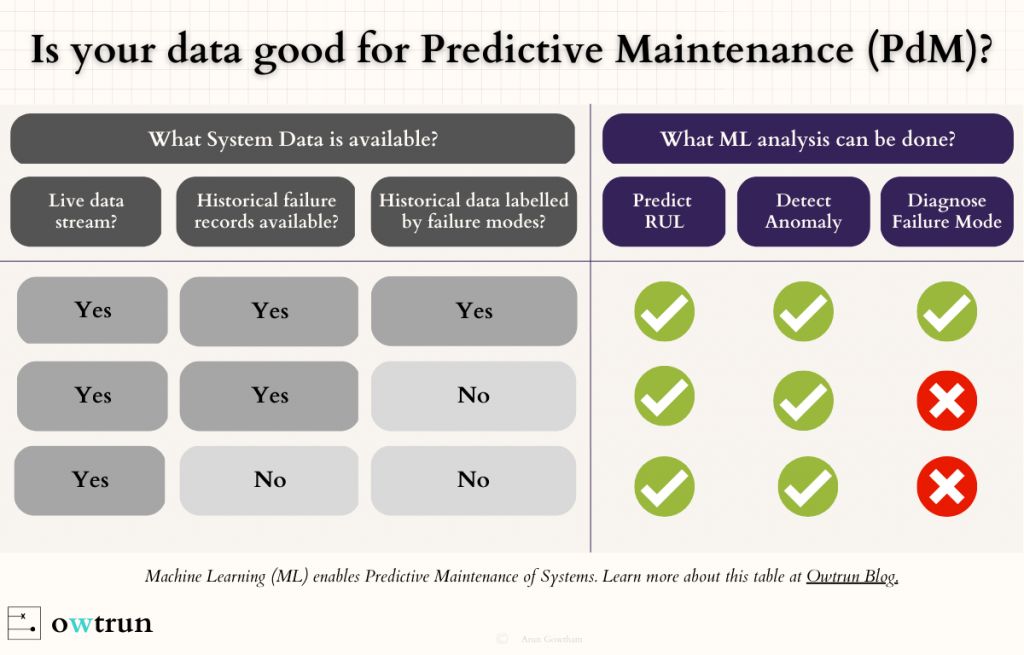
- System – can be a single piece of equipment (asset) or a fleet
- System Data – can be Performance data (Process Temperature or Pressure) or Condition data (Vibration)
- Live Data Stream – can be continuous (time series) or discrete (manual data collection)
- Historical Failure Records – can be complete life data (from normal to failure state) or just time-to-failure data. When no data is available, degradation modeling can be used with thresholds
- Labels – the grouping of failures based on failure modes. Can be just two classes: Normal or Failed State; or multiple classes: Failure Mode A or B or C.
Each of the analyses listed above offers Reliability & Maintenance Engineers the ability to understand the performance and tweak the maintenance plan.
- Predict RUL – Answers the question “How long will the System run before failure?” This analysis predicts the total remaining useful life (RUL) considering the current operating state and historical performance
- Detect Anomaly – Answers the question “What happened?” This analysis is used to monitor the operation of the system to detect deviation from normal performance. All failure mechanisms exhibit a symptom before total failure and this analysis flags this as an early warning
- Diagnose Failure Mode – Answers the question “Why did the failure happen?” Root cause analysis is the most powerful tool in Reliability Improvement and this analysis aids in identifying the most probable cause of anomaly from the similarity patterns of historical data
Given these data requirements for various analyses, the natural next question that arises is “How much of these data is needed? A rule of thumb to start any machine learning algorithm is to have 10x the data size than the number of features analyzed. The primary determinant in deciding what data to use & how much is the Reliability Objective of the analysis. What is the team trying to optimize? How critical is the outcome? How does it impact the business?
We help organizations develop a Digital Asset Management strategy by answering the above questions and seamlessly integrating the solution with the workflow. Contact us to learn more.
Leave a Reply