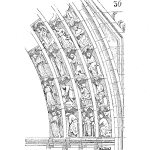
One of the very first plots to do with a string of data is a simple run plot. This plot provides information related to location, trends, patterns, and anomalies, The plot of the data over time is a rather informative chart.
You have most likely constructed many run charts; if not you really should. This short introduction to the run chart will cover creating and interpreting them. Plus we’ll mention a few cautions and tips, as well.
When to Build a Run Chart
A run chart works with a single data variable associated with a time sequence or meaningful order. For example, measuring cut length of nails as they come out of the cutting machine in sequence. Another example would be the monthly water consumption for a household.
On the other hand, if making the same measurement of a random collection of nails. Let’s say they are in a bin and are not organized by the sequence of manufacture, we have a single variable without the time sequence information.
Therefore, when collecting and organizing the data with a time sequence, use a run chart to view the data.
How to Create a Run Chart
After collecting the data, check that the data is in time order. I’m going to plot the monthly water use (I record the water meter reading on the first of each month – I know that month lengths vary a bit, yet I can remember to check on the first of each month). Then calculate the monthly use by taking the difference in sequential months’ readings.
In my data set, the first three readings are 511.11, 514.99, and 518.41. Therefore starting the second month, we can determine the previous month’s use, 514.99 – 511.11 = 3. The next month’s use is 518.41 – 514.99 = 3.42. We have almost five years of data.
Since my data is in Excel, highlight the data column and select View | Insert | Scatter Plot (I’m using just the simple plot using dots – you could also connect the dots, if desired.
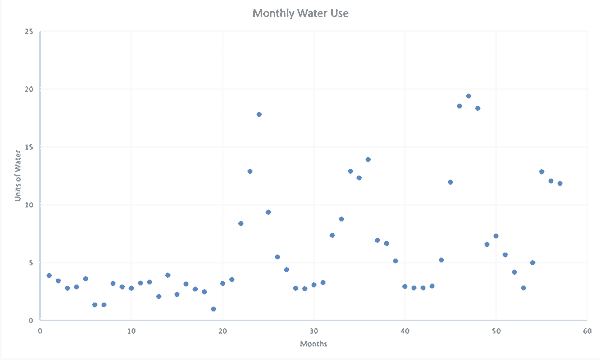
In R, assign the data to a variable, x <- c(3.88, 3.42, … ). Then plot that variable, plot(x). You could also plot the data directly plot(c(3.88, 3.42, … )) if you’d like.
Remember to add a title, axis labels, etc.
Interpreting the Chart Well
On first look, does the chart make sense? Any clerical errors or mistakes in the dataset? Second, what do you and others know about the process being measured? Do the patterns revealed in the plot make sense with what is known about the process? We installed a large vegetable garden and landscaping all around the house – and we did a lot more outdoor watering during the late summer each year.
In the monthly water use run chart, the first 20 months seem consistent between 1 and 4 units of water. Then there seem to be annual spikes in water use each year thereafter.
You likely noticed the change in use and the magnitude of the changes. Also, the increases in water use spike were on an annual cycle. Plus the overall water use is increasing.
Sometimes, the run chart will reveal patterns you did not notice before. Thus, this simple chart will help you learn more about the measured process.
Cautions and Tips
Be aware of the time order or sequencing of the data. If interested in nail cut length and using a box of 100 nails from the hardware store, we may learn about the mean and variance, yet we will not have meaningful information about changes or patterns due to time-related changes in the cutting machine.
Instead, if we record the nail cut length in the sequence of being taken from the box, we may find a time sequence pattern related to our measurement system. Given the nails in the box are in random order and not in the sequence of manufacture.
While creating a run chart is easy, it is informative as related to a time sequence. Therefore, be cautious during data collection and recording to preserve the time sequence information.
A run chart is not a control chart, yet it provides some information related to stability, trends, etc. To determine process stability, consider a run chart may as a quick look, yet a control chart is an appropriate tool to assess stability.
Finally, remember that a run chart may appear to show a trend or pattern, yet it may be due to random fluctuation. Always compare what you see in the plot with what you know (or can verify) about the process under study.
Run charts are one of the 7 Basic Quality Tools (on some lists) and should be a routine tool when examining a time-sequenced variable.
Leave a Reply