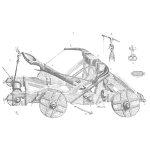
In Part I of this article, we introduced the concept of utilizing Deviation from Nominal (DNOM) control charts for short production runs. These charts allow us to monitor process characteristics over time even when the units being controlled have varying nominal values. DNOM charts assume that the process variability (i.e. standard deviation) does not vary significantly by part type. However, often this assumption does not hold. Characteristics with larger nominal values tend to have more variation than characteristics with smaller nominal values. In Part II we discuss how to test whether or not significant differences in variability exist and if so, how to modify the DNOM methods and charts to handle this situation.
Testing for Variation Differences
A statistical test may be performed to test whether the differences observed in the standard deviations (or variances) of multiple groups represent a statistically significant difference. Statistically significant differences mean that it is very unlikely that the magnitude of the differences occurred by chance.
Recall the data we presented in the example in Part I of the article:
When only two groups are being tested, an F test is performed (if each group follows a normal distribution) or a Levene’s Test is performed (if the normality assumption does not hold).
When more than two groups are being tested, a Bartlett’s test is performed (if the groups follow a normal distribution) or a Levene’s Test is performed (if the normality assumption does not hold).
The above graphic illustrates the results of the hypothesis test for equal variances for the 3 part types shown in the previous data. The relatively large p values (> 0.05) indicate that we do not have sufficient evidence to conclude that the variances are different (with 95% confidence).
In this case, a regular DNOM chart (and S chart) would be appropriate.
Variation Differences
Now, we consider the case where the groups do not share a common degree of variation. Data on Inner Diameter on 3 Part Types is shown below. A statistical test is performed to test for equality of variances.
Clearly more variation exists for the larger diameter parts than for the smaller diameter parts. The very small p-values (< 0.0005) indicate that the differences in group variances are statistically significant. You can also see this by noticing that the estimated standard deviations of each group fall outside the confidence intervals for the other groups’ estimated standard deviations.
What if we hadn’t checked and just constructed a regular DNOM and S chart? Can the 3 part types (segregated by the dashed vertical lines) be adequately monitored on these charts (on the next page)?
Standardized DNOM and Standardized S Charts
Since the control limits are based on the observed variation, we need a method to account for the variation differences between the groups. The solution to the problem is a Standardized DNOM chart (to monitor location) and Standardized S chart (to monitor variation).
The Standardized DNOM chart plots the number of standard deviations each sample average is from the nominal value. This is found simply by computing the deviation from nominal (as before) and dividing it by the estimated standard deviation for that part type. Since each plotted sample statistic accounts for its specific group standard deviation, the data can be combined onto one chart. The Standardized S Chart plots the ratio of the Sample Standard Deviation to the Group Standard Deviation. The following table illustrates the required calculations.
The standardized DNOM is in the next to last column and the standardized S statistic is in the last column. The notation, key statistics, and control limits for both charts are shown below.
Standardized DNOM and Standardized S Notation and Control Chart Calculations
Standardized DNOM and Standardized S charts based on the above data and calculations are shown below.
Summary
This article completes the 2-part series describing control charts for short production runs. This article presented methods to test whether or not significant differences in variability among the part types exist. When significant differences in variability exist, Standardized DNOM and S charts should be utilized.
Leave a Reply