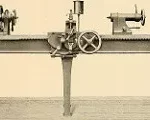
Can You Have a High MTBF and Low Reliability?
As regular readers know, MTBF by itself is misleading. It can also be deceptive when representing actual data. Just because you have a high MTBF value doesn’t mean it is reliable.
In a previous article, 10 Reasons to Avoid MTBF, I mentioned that it is possible to have a relatively high MTBF value when the actual reliability is low. Ashley sent me the following note:
Hi Fred, i love reading your articles they are very informative. I have a question about something you said in a comment which i am hoping you will be able to clarify for me. You said products with higher MTBF can actually be less reliable than products with a lower MTBF
I have tried to find information on how this is possible online, and tried to do the maths myself to make this happen but i have to admit i am struggling.
No worries, Ashley, let’s work out an example to illustrate what I meant.
A Sample Set of Data
Let’s create an example data set with a decreasing hazard rate. I used R and the command of
round(rweibull(10,0.5,500))
This provided a set of 10 values drawn at random from a Weibull distribution with a beta = 0.5 and eta = 500. The values are:
56, 5, 2559, 1147, 486, 931, 1, 1166, 786, 2.
Let’s say this is in hours of operation till failure from a set of 10 motors. We have complete data, no censoring, nice and simple.
The MTBF Value
Let’s calculate the MTBF of these items. You may argue we should calculate MTTF here since we are not repairing the motor, and the calculation is the same.
We would like to know if the measured reliability (MTBF) is below the manufacturer’s claim of 500 hours MTBF, as we are considering buying a new type of motor. These motors are used for 168-hour (1-week) runs, and we’d like to maintain relatively high reliability over 168 hours.
The classic way to calculate MTBF is to tally up the run times and divide by the number of failures. We have a sum of 7,139, and with 10 failures, we estimate MTBF as 713.9 hours. This is above the vendor’s claim of 500, so we are supporting the notion that these are good motors.
The Weibull-Based MTBF
A quick inspection of the data shows a cluster of early failures and quite a bit of time between failures as the equipment ages. There seems to be a decreasing hazard rate at play here; thus, our assumption underlying using MTBF may be suspect.
Let’s fit a Weibull distribution to the data. Firing up Weibull++ and using default fitting for a Weibull 2-parameter distribution, we find beta = 0.39664 and eta = 454.137744. The data has a beta below 1, thus showing a decreasing hazard rate over time.
Using the MTBF calculation based on the Weibull distribution fitted parameters, we determined that the MTBF is 1,545 hours. For details on the calculation, see the article Determine MTBF Given a Weibull Distribution.
Even more evidence based on the data shows that the performance is well above the vendor’s claim of 500 hours MTBF. Let’s double the order of these fine machines.
Let’s Consider Reliability Instead
We run these motors for 168 hours at a time. So, what is the probability that a motor will survive 168 hours once installed?
Using the exponential distribution (MTBF estimate), we find the reliability from time 0 to 168 hours is 79%. Using the exponential reliability function, R(t) = exp [ – t / θ ], here.
A similar question is: What is the chance of successful operation over 168 hours on the 10th time we run the motor (from 1,512 to 1680 hours of lifetime operation or the tenth run)? This assumes the motor has survived through 9 runs. In this case, we find, not surprisingly, that given the assumed constant hazard rate and memoryless property of the exponential distribution, the expected reliability is 79%.
Using the Weibull distribution, we find the reliability from time 0 to 168 hours is 51%, much lower than the estimate based on the MTBF calculation. We could make a decision based on the 1,545-hour MTBF value or the estimate of a 50% survival rate over the first 168 hours. 50% is not high reliability, yet 1,545 hours seems rather high.
The 10th run reliability using the Weibull fit likewise assumes the motor has survived running for 9 runs or 1,512 hours. The reliability over the 10th run is 93%, much higher than the MTBF-based estimate.
Conclusion
The data first suggest that the assumption that the exponential distribution describes the data is not true. Thus, calculating MTBF based on the assumption of a constant hazard rate or the exponential distribution provides a misleading result.
The extra step of estimating MTBF after fitting a Weibull distribution makes the motors appear ‘better’ than the initial estimate. An almost 3x increase in MTBF is due to the slope of the fitting distribution. It is the same data, yet accounting for the decreasing hazard rate results in a higher value for the MTBF. Remember that the MTBF is the mean of the distribution, and a Weibull distribution with a beta of 0.5 is heavily right-skewed. (Long tail to the right…)
Based on the Weibull, it suggests that some of the motors would run for a very, very long time without failure, even though more than half fail rather quickly.
The reliability estimate depends on the time frame of interest. For the exponential distribution fit, the reliability over 168 hours is 79%, while over 1,680 hours (ten runs), it is 9.5%. For the Weibull distribution fit, the reliability over 168 hours is 51%, and over 1,680 hours is 18.6%.
The bottom line is that using just MTBF, we would buy more of the same motors and ‘enjoy’ the experience of about half the motors failing within their first week of use.
Do you have an example that shows just how badly using MTBF misleads you and decision-makers? Send it over, or add a comment below.
Fred,
Had a great example of this in http://nomtbf.com/2012/06/the-worst-reliability-requirement/.
Very nice and educational text. I have a question: What is the phisical meaning of the characteristic life (eta)?
Sorry, but I put my e-mail address wrongly.
My question is: What is the physical meaning of the characteristic life (η)
Hi William, just as the mean is the center of mass of a normal distribution, or any distribution, the Weibull parameter eta often called the characteristic life is the point in time corresponding to 63.2 percentile of the distribution. It means that roughly 2/3 of the failures occur by that point in time.
Think of a way to define a line, all you need is a slope and a point. For the Weibull distribution the slope is the beta parameter and describes the rate of change of the hazard function. The point is the characteristic life, defined at the 63.2 percentile point.
Physically, it doesn’t have any meaning relative to specific failure mechanisms.
I saw it once, the derivation of the exponential family, which includes the Weibull distribution. The 63.2 percentile falls out of the derivation and if I recall correctly has something to do with the exponential element… recall that e^(-1) = 0.368 roughly.
hope that helps.
Cheers,
Fred
Yes, thank you Fred.
Great learn, thanks Fred!
Following I would plan my operation of Rel evaluation program:
– Identify my gate (baseline), in your case would be 168 hrs.
– Data set sorting: (56, 5, 2559, 1147, 486, 931, 1, 1166, 786, 2), any failures below the gate would be picked: 56,5,1,2, FR already 40%, project failed and defects send back to vendor for FA, I want the RCA with correct action approved.
– Fitting with Weibull only for rest of data, deliver a baseline requirement to compare with later. (I personally do not believe on constant FR for all kind of materials, so….Weibull)
– Vendor re-apply and repeat program until 0% FR below 168, then let’s discuss the price…
The gate of baseline should came from VOC, on top of reliability, let’s consider business instead as well.
Hi Fred,
Hope you are doing good
very nice article Sir.
I am not able to understand this line written in article, “A similar question is what is the chance of successful operation over 168 hours the 10th time we run the motor (from 1,512 to 1680 hours of life time operation or the tenth run).”
My doubt is 10th time we run the motor that means only one motor is being tested and failure is checked
But in earlier case we have taken 10 motor failure.i.e.”6, 5, 2559, 1147, 486, 931, 1, 1166, 786, 2. Let’s say this is in hour of operation till failure from a set of 10 motors. ”
then how can we compare these two.
Hi Piyush,
I should have stated the question as a conditional probability. If the motor runs for 9 cycles of running for a week, 168 hours each week, and survived, what is the chance it will survive over the next cycle (168 hrs) after not failing for the first 9 cycles?
Does that help?
Cheers,
Fred
Hi Fred,
Hope you are doing good
very nice article Sir.
I am not able to understand this line written in article, “A similar question is what is the chance of successful operation over 168 hours the 10th time we run the motor (from 1,512 to 1680 hours of life time operation or the tenth run).”
My doubt is 10th time we run the motor that means only one motor is being tested and failure is checked
But in earlier case we have taken 10 motor failure.i.e.”6, 5, 2559, 1147, 486, 931, 1, 1166, 786, 2. Let’s say this is in hour of operation till failure from a set of 10 motors. ”
then how can we compare these two and i am not able to understand meaning of 10 run Is it run of 10 different motor or single motor. if it is of single motor then one motor is running for 1680 hrs and if it is for 10 different motor that means single motor has not accumulated more than 168 hrs.
kindly clear my doubt.
Thanks,
Piyush
Hi Sir,
That means only one motor is being tested if its like that how and why commutative hrs of 1680 is referred?
Thanks
Piyush
Hi Piyush – let’s say we have a motor, we did prior testing on another batch of motors and have some data.
Now, let’s say we install this new motor and it runs without failure for 9 cycles, 9 x 168 hours as each cycle is a week. All good, and we have a a motor that is 9 x 168 =1,521 hours old.
Great, so the question is what is the probability the motor, that is 1,521 hours old, what is the probability it will run without failure for the next cycle of 168 hours?
Cheers,
Fred
Hi sir,
Thank you very much.
Now its clear.
Thanks,
Piyush
Good morning Fred. I have interests in aircraft maintenance though I’m not a technical person on this field. I want to understand what the general implications of the following is:
1.) High and low Removal Rate of aircraft components
2.) PIREPS analysis showing figures of components below the Calculated Alert Rate
3.) Relationship between Alert Rate and Exceedence Rate
Your kind explanation of these in simple terms will be very much appreciated.
Thanks
Hi Enock,
I’m not that familiar with the aircraft maintenance industry and am unable to answer your questions. maybe someone else that reads this blog will be able to comment.
Cheers,
Fred