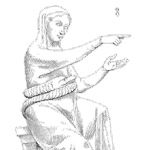
Historically Reliability Engineering of Electronics has been dominated by the belief that 1) The life or percentage of complex hardware failures that occurs over time can be estimated, predicted, or modeled and 2) Reliability of electronic systems can be calculated or estimated through statistical and probabilistic methods to improve hardware reliability. The amazing thing about this is that during the many decades that reliabilityengineers have been taught this and believe that this is true, there is little if any empirical field data from the vast majority of verified failures that shows any correlation with calculated predictions of failure rates.
The probabilistic statistical predictions based on broad assumptions of the underlying physical causes begin with the first electronics reliability prediction guide begin November 1956, with the publication of the RCA release TR-1100, “Reliability Stress Analysis for Electronic Equipment”, which presented models for computing rates of component failures. This publication was followed by the “RADC Reliability Notebook” in October 1959, and the publication of a military reliability prediction handbook format known as MIL-HDBK-217.
It still continues today with various software applications which are progenies of the MIL-HDBK-217. Underlying these “reliability prediction assessment” methods and calculations is the assumption that the main driver of unreliability is due to components that have intrinsic failure rates moderated by the absolute temperature. It has been assumed that the component failure rates follow the Arrhenius equation and that component failure rates approximately doubles for every 10 °C.
MIL-HDBK-217 was removed from the military as reference document in 1996 and has not been updated since that time; it is still being reference unofficially by military contractors and still believed to have some validity even without any supporting evidence.
Much of the slow change in the industry is due to the fact that electronics reliability engineering has a fundamental “knowledge distribution” problem in that real field failure data, and the root causes of those failures can never be shared with the larger reliability engineering community. Reliability data is some of the most confidential sensitive data a manufacturer has, and short of a court order will never be published. Without this real data and information being disseminated and shared, one can expect little change in the beliefs of the vast majority of the electronics reliability engineering community.
Even though the probabilistic prediction approach to reliability has been practiced and applied for decades any engineer who has seen the root causes of verified field failures will observe that most all failures that occur before the electronic system is technologically obsolete, are caused by 1) errors in manufacturing 2) overlooked design margins 3) or accidental overstress or abuse by the customer. The timing of the root causes of these failures, which many times are driven by multiple events or stresses, are random and inconsistent. Therefore there is no basis for applying statistical or probabilistic predictive methods. Most users of predictions have observed the non-correlation between estimated and actual failure rates.
It is long past time that the electronics design and manufacturing organizations to abandon these invalid and misleading approaches, acknowledge that reliability cannot be estimated from assumptions and calculations, and start using “stress to limits” to find latent failure mechanisms before a product is released to market. It is true that you cannot derive a time to failure for most systems, but then no test can provide an actual field “life” estimate for a complex electronic system nor do we need to. There is more life than needed in most electronics for most applications.
Fortunately, there is an alternative. A much more pragmatic and effective approach is to find to put most engineering and testing resources to discovery of overlooked design margins or a weakest link early in the design process (HALT) and then use that strength and durability to quickly screen (HASS) for errors during manufacturing. HALT and HASS have little to do with a specific type of chamber or chamber capabilities. It is a fundamental change in the frame of reference for reliability development, moving instead from time metrics to stress/limit metrics. Many have already realized this new frame of reference. Since they have found these methods much more efficient and cost effective for developing robust electronics systems, it gives them a competitive advantage. They are not about to let the world or their competitors know of how successful these methods are.
Great little article, Kirk.
I wish some Reliability Engineers would start to assimilate more rather than just take the monkey-see monkey-do approach.
Thanks Dave, since real reliability issues are not generally published, the monkeys will keep doing the same thing and wasting lots of money and time doing it (predictions).
You’re right; I seldom find installed base and failure counts any more. Medtronic used to publish Pacemaker sales and failure counts, so I used their counts data to estimate nonparametric field reliability and failure rate functions and compare them with Medtronic’s own field reliability estimates based on observed times-to-failures. So Medtronic quit publishing Pacemaker sales and failure counts.
“MIL-HDBK-217G (George),” Reliability Review, ASQC, Vol. 12, No. 3, June. 1992 makes MTBF predictions under the constraint that predicted Paretos agree with observed (field) Paretos.
I haven’t updated that because there’s a better way: https://sites.google.com/site/fieldreliability/credible-reliability-prediction based on the observations that generations of products or parts have proportional failure rates, because they have similar designs, use similar or same parts, and have same customers and environments.
Why not combine testing with field reliability data? FDA allows Phase II clinical trials life tests to use population life statistics.
Thanks Larry for your comments and link.