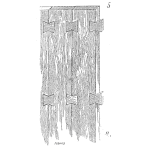
Ergodicity means that cross-section probabilities equal longitudinal lifetime probabilities. (“Ergos” is Greek for “work.” Think of “ergonomics”.) Ergodicity means that we can estimate age-specific field reliability functions from cross-section data: ships (installed base) and returns (complaints, failures, service parts’ sales, etc.). Ships and returns provide information about lifetimes. Returns are the superpositions of failures of products or their parts started at different times. What does ergodicity have to do with toilet paper?
Ergodicity in action
My wife says the toilet paper roll must be mounted so the paper rolls off the outside. In my carefree days as a janitor, I never realized that! Is that a fact known only to women? Ergodicity says I could test the hypotheses that is a fact known only to women,
Ho: P[Rolls off outside|women} = P[Rolls off outside|men} vs.
Ha: P[Rolls off outside|women} > P[Rolls off outside|men},
by observing the company’s women’s and men’s toilets over time to record the direction the toilet paper rolls off whenever it is replaced. (We have a self- service system.) However I can’t continuously observe the women’s toilets, so I’ll observe all of the women’s toilets at 3 AM and estimate the proportion that have the toilet paper rolling off the outside. The proportion over all women’s toilets from one cross-section observation should approximate long run proportion on women’s toilets. Does that motivate you to consider cross-section data for reliability information?
Conditions for Ergodicity
Stationarity in mean and in autocovariance or autocorrelation characterize ergodicity. Imagine a steady state stochastic process of ships and returns long after its start. The original cohort is probably all dead and returns come from different past cohorts, and there would be no way of telling. But what if you observe an ergodic process from its beginning or over some time?
Suppose you have cohorts of vehicle counts that use the auto parts and observe monthly parts’ sales for a couple of years. That’s enough to make nonparametric estimates of parts’ reliability for as old as the oldest part in the vehicle installed base.
I apologize for not proving the identifiability of a reliability function from ships and returns counts. I regard times-to-failures as outputs of an M/G/infinity self-service system. The fact that one can write the likelihood function https://fred-schenkelberg-project.prev01.rmkr.net/?s=entropy is evidence that ships and returns counts contain reliability information. The article by Kielson and Servi states that “Networks of M/G/infinity queues with probabilistic routing are BCMP networks with simple known ergodic distributions.“ (BCMP are initials of four authors whose article defines conditions for ergodicity of queuing networks. https://en.wikipedia.org/wiki/BCMP_network)
The Kielson and Servi article extends that claim to nonstationary Poisson input M(t)/G/infinity self-service systems. (It’s difficult to disprove a sequence of independent ships observations are nonstationary Poisson.) The population in an M(t)/G/infinity self-service system is the input minus the output, so observing inputs and outputs identifies the population remaining in the system and their waiting times. Waiting times are the life times of products or their service parts.
Suppose you count only the starts of life (ships) N1 at time t1 and count, at later time t, how many returns R(t), without identifying each one. These counts would give an estimate of reliability: R(t-t1) = R1/N1. Suppose you had more ships, N1, N2, N3,… and more returns at several times as in figure 1.

Suppose you observe ships cohorts N1 and N2 from times t1 and t2 and returns R1 and R2. Returns R2 at time t could have come from cohort N1 with probability p2 (reliability R(t-t1)) or from cohort N2 with probability p1 (reliability R(t-t2). A geophysicist masquerading as an Apple reliability engineer claimed, “It’s too hard to solve for those probabilities.” Maximum likelihood and least squares provide methods for estimating those probabilities and nonparametric reliability functions. You could use the maximum likelihood estimator spreadsheet, npmle.xlsx, https://sites.google.com/site/fieldreliability/home/files-workbooks-etc. Or read the next article in “Progress in Field Reliability?” series on least squares nonparametric estimation.
References
Billingsley, P. (1965). Ergodic Theory and Information, Wiley, New York
Kielson and L. D. Servi, “Networks of Non-homogeneous M/G/∞ Systems, DTE Laboratories, Dec. 1989
Leave a Reply