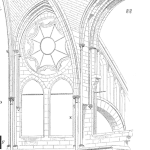
When processes trend naturally due to tool wear, traditional control charting methods fail. The trend (which is expected) results in inappropriate “out-of-control” signals. Control charts should detect unexpected changes in the process. If the trend is expected, we do not want to be alerted to this trend. If no accommodation is made for this trend, the chart will incorrectly produce “out-of-control” signals.
The chart below illustrates a traditional X-bar chart on a trending process characteristic.
Setting up charts for trending data requires that we first understand and model the trend that naturally occurs. That is, we need understand how our measurements change naturally over “time” where “time” is the variable that correlates with the trend (such as production minutes, hours, cycles, punches, parts produced, etc.).
If the trend is linear, simple linear regression may be used to develop a simple linear model to describe and predict the mean value over “time” (i.e. the trend). Non-linear trends will require more complex models. The graphic below illustrates a simple linear model fitted to the data. The process mean is predicted as a function of “time” and only values that are acceptable should be included (values spanning the range from a new tool to a tool just prior to it being replaced).
The model is: Mean = 7.668 + 0.1020*Time and it appears to fit quite well over the range of responses included.
Once an adequate model is developed to describe the mean value, control limits may be specified that are parallel to the process average line. If we are charting averages, then typically the control limits will be parallel to the mean trend line at a distance of +/- 3 standard deviations.
A control chart that incorporates the trend is shown below. Only averages that deviate more than the expected trend should be considered a signal of special cause variation.
Now, although the above chart is technically correct, it may be confusing since the control limits are not horizontal. To resolve this, a control chart may be constructed on the differences between the actual sample average and the predicted average for each sample. Note that the predicted average depends on the model we found earlier and the control limits must still reflect the within sample variation. This chart is shown next.
In effect, we have simply rotated the trending chart and established the centerline at zero. As mentioned earlier, non-linear trends may be modeled if necessary, but more complex models are required.
Leave a Reply