
Reliability, Availability, and Maintainability (RAM) modeling overview
The fundamental purpose of Reliability, Availability, and Maintainability (RAM) modeling is to quantify system performance, typically in a future time interval. A system is a collection of items that operate together to produce an output, often a production value. These items can include subsystems, components, software, human operations, and more. For example, an automobile can be viewed as a system with subcomponents like the drive train, engine, gearbox, etc. In RAM models, it is crucial to consider the relationships between these items to determine the system’s final output. They have proven effective in various industries as tools for cost avoidance, decision-making, and validating assumptions made by internal stakeholders.
Building the model and its granularity level
Building a RAM model requires inputs such as equipment performance records, operating philosophy, operating costs, and the desired production throughput. The model is typically run over a future time interval, (e.g. five years), and provides valuable information to the operator. This information can include the total cost of operating the system, production losses, spare parts usage, as well as the impacts of weak links or bottlenecks in the system. For example, analyzing spare parts usage requires incorporating the components associated with those spare parts into the model. As the model’s level of detail increases, the number of blocks in the model also increases as shown in the diagram below. For instance, starting at the top of the diagram, the System Level Process Unit 2 block can be broken down into a 4-block pumping Sub System (Sub System Level 1), and the Pump element can be further divided into 5 blocks, as shown in Sub System Level 2. Consequently, if the motor has 5 subcomponents and each valve has 3 subcomponents, Process Unit 2 alone would become a 17-block system.
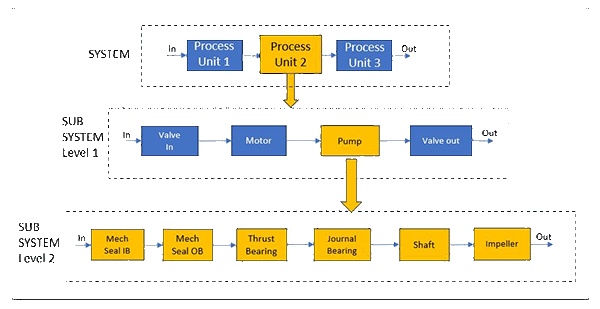
Advantages and drawbacks of granularity
Increasing granularity provides more detailed and precise information about the system’s overall performance over the desired future time interval. Each block contains specific information, particularly regarding life distributions, costs, and maintenance tasks. Therefore, processes like maintenance strategies can be detailed, and any necessary improvements to block performance can be quantified and justified. However, granularity also has its drawbacks, which include the following:
- More blocks necessitate more information and analysis for each block, ultimately increasing the time required to build the entire RAM model.
- Similarly, more blocks require more time to run the model. RAM models with a large number of blocks (over 1,000) and complex operating philosophies may take days (Yes! Multiples of 24 hours) to run.
Ultimately, the trade-off depends on what the operator requires from the model. For instance, if the goal is to identify weak links in the system, an equipment model like Sub Component Level 1 in the diagram above would suffice. On the other hand, determining spare part min/max levels in a warehouse would necessitate a more granular level (Sub Component Level 2). The complexity of the model should be based on the asset’s cost and criticality. For example, an orbital satellite system, which is expensive to build and launch usually requires high reliability due to the lack of possible human intervention once in space, would benefit from more granular level models.
RAM models are flexible and can be easily modified. In cases where a mixture of critical and non-critical subsystems exists, a combination of Sub Component Level 1 and Level 2 can be built. As mentioned before, the key is to identify the needs and objectives of the operator and understand how the system functions before deciding on the model’s complexity and level of granularity.
Leave a Reply