
Guest Post by James Kline (first posted on CERM ® RISK INSIGHTS – reposted here with permission)
Dianna Deeney in her piece in CERM Insights #362 notes that big data may provide the quality profession with more professional opportunities. Dr Anil Maheshwari in his book Data Analytics: Made Accessible specifically indicates that quality management will benefit from big data. (1)
There can be no doubt that big data is an important environmental change and challenge for most businesses. It changes the environment because with increased computer storage and computational power, companies can access and evaluate more and more information about process performance and customer satisfaction. It is a challenge because the field is relatively new, and management is still trying to understand how the information can best be applied.
It is worth assessing the basic composition of big data. The skills needed to successfully analyze and present big data. And how these skills compare with the skills required for a Six Sigma Black Belt. The black belt skills are used for two reasons. First, it is among the highest and most sophisticated certification in the quality field. Second, big data analysis requires the use of sophisticated statistical and mathematical techniques, as well as, computer skills. Such a comparison will provide insights into how well a quality professional will be able to adapt to the big data environment.
Big Data
Big data is as the name implies, a lot of data. By a lot, I mean a humongous amount of data. Big data is generally divided into two broad categories. These are structured and non-structured. Structured data is data that is codified. It includes statistics, tables, the content of notebooks which have been digitized, or names and addresses. Unstructured data includes photographs, videos, and tweets. (2)
The methods used to analyze structured data have been well developed over time. However, unstructured data analysis requires the development of additional tools. Further, because of the size of the data that is available for analysis, methods had to be developed to store, sort through, and analyze the data that is relevant.
Some of the skills needed to sort through and analyze big data are like those used by existing statistically sophisticated positions, such as the Six Sigma Black Belt. But there are also significant differences. These differences have resulted in the creation of data analyst positions within almost all economic sectors.
To demonstrate this, I pulled, from the internet, a typical assessment of what a data analyst does and the fundamental skills they need.
Data Analyst Duties and Skills
Succinctly put, a Data Analyst collects and identifies the relevant data. Performs quality assurance on the data. Analyzes and presents the results. The most common hard skills needed are.
- Data Collection
- Statistical Modeling
- Data Mining
- Data Base Management
- Report Generation
The entry level position requires a Bachelors in Math, Statistics, Economics, Finance, Computer Science, or background in the specific organization’s economic sector. Skills using SAS, Excel, Power BI and Tableau, Python, R, SQL, are also desired. For higher level positions a Master’s, or PhD in computer science, mathematics, or statistics is desired.
From this brief presentation, one can see that data analysts have a specific skill set. This skill set is different from that of a typical quality professional. This can be demonstrated by examining the specific statistical tools used by both the data analyst and the Six Sigma Black Belt. (The Black Belt statistical tool are obtained from the Six Sigma Handbook (3))
Comparison of Statistical Methods
The Table below is a comparison of the statistical skills associated with Data Analyst and Black Belt positions.
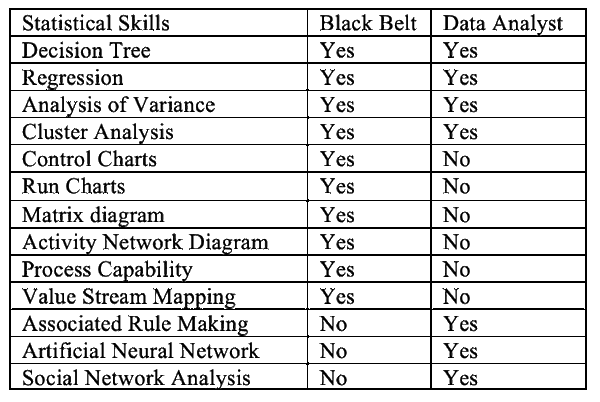
While the listing of statistical skills is not extensive, despite overlaps, there are fundamental differences. Black Belts focus on process analysis, while Data Analysts focus on separating data sets and evaluating nonstandard data.
Paul Gladieux in his CERM Risk Insights #319-piece notes:
“.. proficiency in the profession mandates a working knowledge of the language which over the past one hundred years has resulted in a vocabulary of over 500 quality-related terms listed by the ASQ.”
The same is true for the vocabulary of the big data professionals. That means that as big data increasingly imbeds into the quality field, quality professionals will have to learn new skills and vocabulary. Further, the quality professional will need to beef up his/her computer skills. They will have to know at least some of the following – Power BI and Tableau, Python, R, or SQL.
The question is: If there is a tug of war between the two professions, which professional skills are going to dominate? My guess is the big data’s. This is because their required skill set encompass many the Six Sigma Black Belt statistical skills. While Six Sigma Black Belts do not have the knowledge base to manage the non-structured data nor the computer programing skills required of the data analyst.
Conclusion
Big data is a concept and process which is gaining more and more traction in companies worldwide. While it offers opportunities for quality professionals, there are substantive difference between the requirement of the two professions. As big data becomes more intrenched in organizational activities, its skill set requirement could edge out those currently required of quality professionals like Six Sigma Black Belts.
If professional level certifications like Six Sigma Black Belts are going to remain relevant in an era of big data, the body of knowledge will have to be adjusted to accommodate this new environment.
Endnotes
- Maheshwari, Anil, 2022, Data Analytics: Made Accessible, available on Amazon
- Holmes, Dawn E., 2017, Big Data: A Very Short Introduction, Oxford University Press, Oxford U.K. page 5.
- Pyzdek, Thomas, 2003, The Six Sigma Handbook, McGraw-Hill, New York N.Y.
James J. Kline has a PhD from Portland State University. He has worked for federal, state, and local government. He has consulted on economic, quality and workforce development issues. He has authored numerous articles on quality and risk management. His book “Enterprise Risk Management in Government: Implementing ISO 31000:2018” is available on Amazon. He can be contacted on LinkedIn or jamesjk1236@outlook.com
Bio:
James J. Kline has a PhD from Portland State University. He has worked for federal, state, and local government. He has consulted on economic, quality and workforce development issues. He has authored numerous articles on quality and risk management. His book “Enterprise Risk Management in Government: Implementing ISO 31000:2018” is available on Amazon. He can be contacted on LinkedIn or jamesjk1236@outlook.com
Leave a Reply