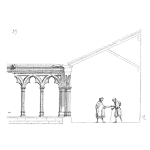
There’s a quote one of my previous managers had in his cubicle: “Being a manager is easy, it’s like riding a bike. Except that the bike is on fire, you’re on fire, and everything is on fire”. I chuckle every time I read it and then reflect upon the reality of dealing with project deadlines, deliverables, and performance. Reliability Engineering & Asset Maintenance initiatives have a project management aspect to them.
They need to prioritize tasks, invest smartly, balance resources, and manage timelines within business operations. “Reliability Management” as a subject area grew to address this specific challenge.
Adding AI into this tussle can make or break a program. AI-based projects (which applies equally to Machine Learning/ IoT projects) are multi-disciplinary in nature and require collaboration among various technical teams for successful completion. This complexity adds fuel to the fire. Here are some of the ways to douse it and lead a successful project:
1. Align Project goals with Business goals
AI solutions’ versatility is a double-edged sword. It can be applied to many processes within a business, but it should not be applied everywhere; for it can dilute the efforts and distract the team. Select a project and establish its objectives that match the higher-level business goal. The article titled “Under the Double Umbrella” talks more. If the business goal is to increase the Availability of a system by x%, then a sample Predictive Maintenance project can be chosen to plan maintenance & must report its performance in the same metric.
2. Structure teams for success
Assess your own organization’s skills with a radical openness to carry out a project of this scope. Firms sustain on the strength of their processes and SOPs. These ways of working have a cultural impact on employees and they can impede the introduction of new technology. Studies have shown that creating a new team with diverse technical experts will increase the chances of success. This special team can be structured outside the bounds of regular rules, so they have the freedom to utilize resources and move swiftly in execution.
3. Parallel Participation
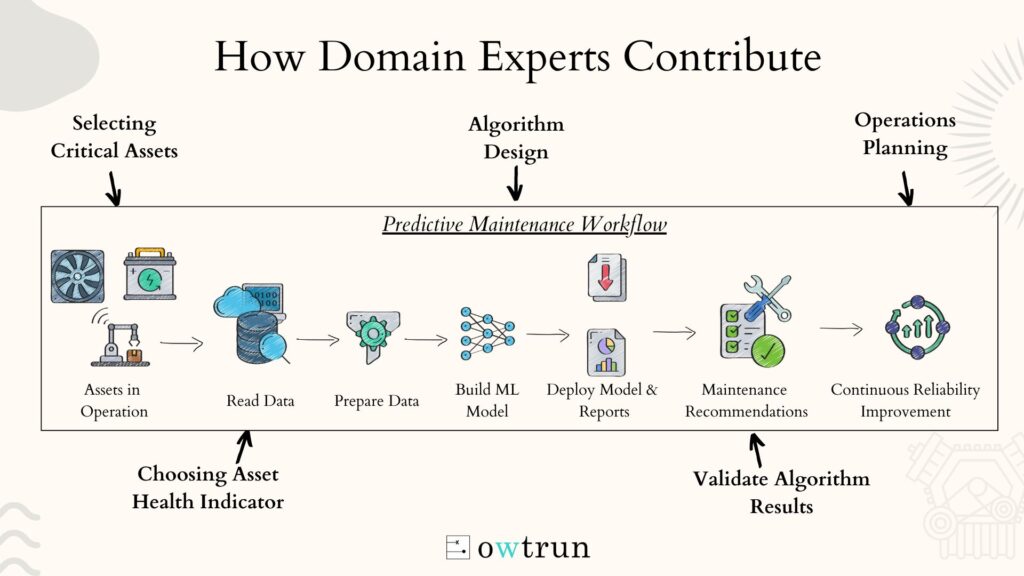
Domain experts should be involved with the special teams to design & execute the AI project. Not considering the expertise of maintenance technicians in planning a Predictive Maintenance solution is one of the glaring errors cited in PdM research for its low success rate. Another advantage: soliciting feedback from end-users who would be impacted by the project will also make them feel appreciated and speed up the acceptability of a new solution.
Part -2 of this article covers three more tips for successfully managing AI-based projects in your organization.
Leave a Reply