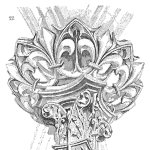
The concept of degradation variables
Assets typically age over time, leading to degraded performance and loss of function. Asset life models are built in order to predict future degradation patterns. Those models are based on asset degradation variables such as time or usage. Those variables could be for example, time between failures or distance covered between failures. Many assets have more than one degradation variable. In this case, it is important to define which of the multiple variables is the dominant one and will subsequently provide the Reliability Engineer with the most precise life model.
Reliability is a probability. Specifically, the probability that a system will perform its intended function within a specified mission time and under specific process conditions. Therefore, most reliability calculations incorporate a time element as a degradation variable. Generally, when building life models, we default to using calendar time as it is more straightforward. We have had tools to easily measure elapsed calendar time for centuries now.
Issues with using calendar time as a degradation variable
However, using calendar time to evaluate aging or any degradation variable might be incorrect for many assets. Some assets may remain idle for part of the time, thus delaying degradation mechanisms, such as mechanical wear. A typical example is an automobile which spends a large part of the year sitting idle in a garage. When the automobile is used, the aging process occurs through wear and tear of various components. The aging of the automobile and subsequent likelihood of failure increases as it is driven. In this case, a different degradation variable comes into play: the time the automobile is running, also known as “operating time” or “run time”. Operating time is logically shorter than calendar time. This highlights the potential exaggeration of timelines if calendar time is used. For example, for the same automobile, we would refer to “actual operating time” between failures or between maintenance.
In addition to run time, other degradation variables can be used to evaluate the same automobile, such as the odometer reading. Using odometer readings moves us from a “time-based” measure to a “distance-based” measure. Furthermore, considering how an automobile functions, we can also use the “ignition” or “start-up” frequency. When the automobile is started, significant torque is put on the engine leading to wear and tear over time. In this case, we would refer to a “cycling” measure, relating to the on/off cycle of the automobile. The degradation variable would be “cycling frequency” between failures in this case.
As demonstrated above, we have identified four different degradation variables for an automobile, each contributing to a different life model. The question then arises: which degradation variable leads to the most accurate life model for decision-makers? In other words, what is the “dominant” degradation variable? In practice, if we were able to measure all four variables, we would build four life models for the aforementioned automobile. For each distribution, we would then test the distribution parameter bounds using a Maximum Likelihood estimator and the derived covariance values. This test would then provide us with the dominant variable. Once identified, the dominant variable would be used to build the life model that best represents the automobile’s life characteristics. Consequently, all reliability engineering calculations would be based on this dominant variable-based model.
Challenges with obtaining degradation variables in the real world
However, in practice, we do not measure all degradation variables for an asset. Calendar time and odometer readings are easily obtained using the automobile example mentioned earlier, However, we rarely record driving time or engine cycle time. Additional measurement devices would need be set up to record those last two variables. This limitation also applies to industrial operations, where operators chose to record only a few operating parameters. Some equipment assets such as larger electric motors, come with standardized operation measuring devices. These measuring devices can record on/off cycles via PLC systems, making it easy to obtain actual run time and start/stop cycles for pumps and motors. Note that if a pump is connected to a motor, the run time of the motor is equal to that of the pump. Therefore, the run time to failure of a motor is the same as that of the attached pump. Other types of equipment pose greater challenges in terms of defining an appropriate degradation variable. For instance, valves that open and close do not have an obvious degradation variable. Failure modes for valves could be related to seat or stem damage due to fluid erosion for example. In such cases, consultation with subject matter experts is crucial to determine the correct degradation variable(s) and the most appropriate measuring device(s) to install.
In summary, it is important to identify the appropriate degradation variables for critical assets in an operation. Once identified, if possible and cost effective, measuring devices should be installed in order to record those variables. In the case where there is more than one degradation variable for an asset, the dominant variable needs to be identified in order to obtain the most accurate life model. This will assist in obtaining the optimal maintenance strategy for the asset in question.
Leave a Reply